Reading
A Tale of Two Biases: How To Read The News
Counter two frequent biases while reading the news: Selection Bias and Description Bias.
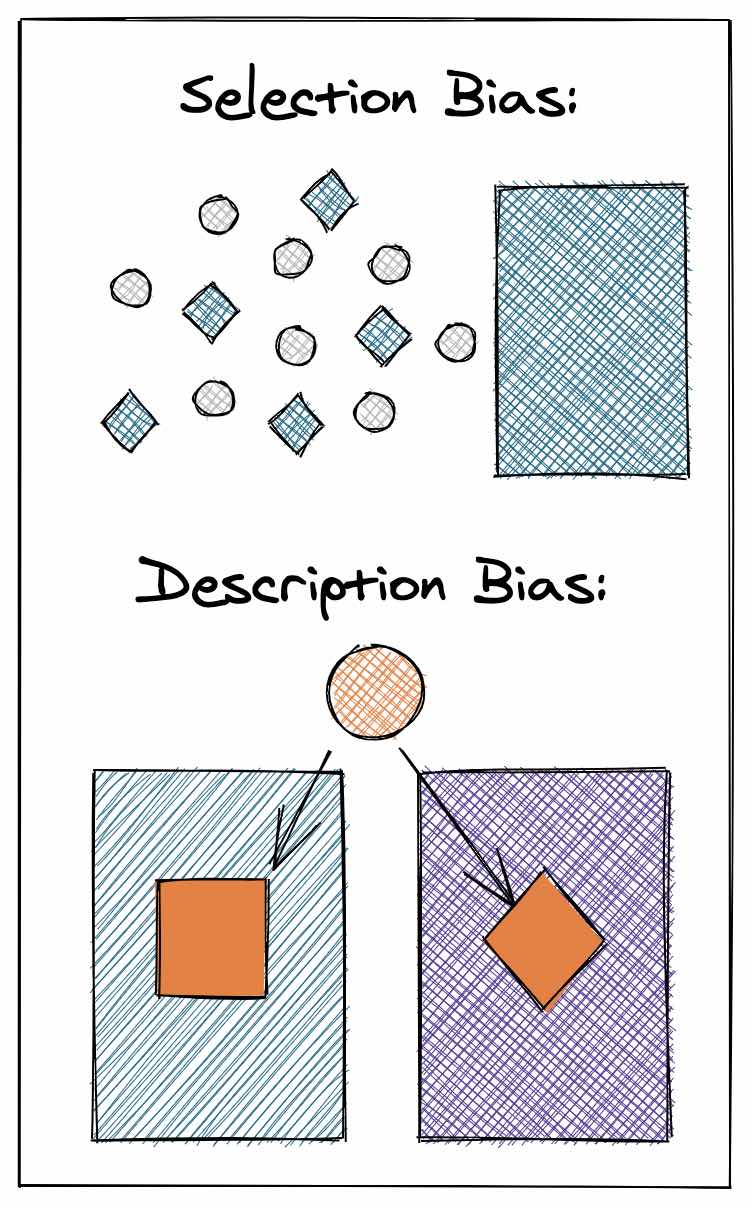
A lot of my early reading during my PhD studies revolved around understanding collective action, and protest in particular. From that reading I have a two insights I want to share with you about biases in news coverage. These biases are not malicious biases in the sense of secret cabals influencing what gets published and what doesn't – they are just descriptions of how news work, and what you have to take into account when you're reading.
To teach you about these two biases, we're going to adopt the viewpoint of a researcher who wants to understand protest quantitatively, and comparatively. How much protest is there, and where does it occur? How do cities and countries differ in how much protest there is? Are there different periods where there's more protest? Do people protest more when the weather is nice compared to when it rains a lot?
Any answer to these questions requires you, the researcher, to know how much protest there is in the first place. But how do you know that? Is there a central protest registry where you can look that up? No, of course not. Maybe you could find a way to have everyone who organises a street protest message you that it's happening – but even if you could achieve that, you'd still be constrained to protests happening from now on...you would not be able to look back and see how protest behaved in the past.
For decades, social scientists have therefore relied on news reports about protest. Thousands of undergrad research assistants have read millions of newspaper articles to then record in Excel files the date and size and other information about protests (in fact, I wrote software that made that a nicer experience than typing into Excel files).
Now, news reports are a fantastic resource for scientists, because they usually have archives going back decades, which makes it easy (well, relatively) to look at protest many years back. When you use news agencies like Reuters, Agent France Press or the BBC, you also get really good international coverage of events, which makes studies comparing protest in different countries possible.
But, despite how useful news reports are, you still have to ask: do the BBC, Reuters or AFP report all protest? Do they report literally every protest that happens in a country?
Selection Bias
Sadly, they do not. Which leads you, the researcher, to ask: okay, then which protests do they cover and which ones don't make it into a report? The problem is: how do you know? You only have the ones they report on, you don't have the actual, ground truth numbers! This question, which protests make it into the news and which don't, is what's called Selection Bias.
Countering selection bias is hard. One thing you can do is look at different sources and compare which protests make it into BBC, Reuters and AFP and which ones make it into only one of them. For these three, the overlap is actually pretty significant, they don't vary much and are thus considered fairly neutral.
Other news sources can differ though, of course. The local newspaper in my town might write a story about a protest happening here that your local newspaper probably will not cover. The protest in my town might also not appear in the feed of the BBC for the same or different reasons: if a BBC reporter is hundreds of kilometers away, they might not hear about the protest or might not be able to make it. And maybe the protest is not considered important: local kids demonstrating against their skate park being torn down might not be considered "news worthy".
Unless there's a huge national discussion going on about kids and fitness, in which case someone might decide "hey, this relates to a wider national discussion going on right now, send the reporter in even if it's far away". Which means the selection process for which protests get covered might differ at different times.
It's clear why that is a problem for you as a researcher, right? If you're interested in how protest influences the national discourse on a topic (was protest successful in changing something?), now you have the national discourse influencing how much protests on a given topic are reported, which biases your results.
So what's the takeaway on selection bias for you, the not-actually-a-researcher-of-protest?
You don't need to go out and compare different outlets in detail for what they cover and what not. But as you're reading the news, ask yourself:
- What is the likelyhood of this particular event being reported a year ago vs. now?
- What is the likelihood of this particular being reported a year from today vs. now?
- Are there other, similar sources that report the same thing?
Now, let's say you have a number of reports from different sources, all covering the same protest. Most of the time there's a bare minimum of information that all sources get right: the date a protest occurred and where it occurred. Anything else gets contentious really fast: how many protestors were there? If you listen to the protestors it's often many more than what the police are reporting (the protestors want to signal lots of people share their viewpoint, the police wants to signal the opposite). Who was protesting, and why?
Description Bias
Here, the second bias comes into play: Description Bias
For description bias, there are basically two reasons: the reporter does not know all the facts or they are "telling a story" – they impose their own viewpoint on what they are reporting. Again, this doesn't have to be malicious and more often than not is simply a consequence of human nature or a selection process. When a newspaper or stations reports on events in a certain way it's less often a consequence of explicit editorial command than an artefact of the editors hiring people that think a certain way.
Not knowing/reporting the facts is, I think, obvious, so let's look at an example of description bias that relates to "telling a story". You, the researcher, want to code news reports of protests in country A, and you encounter the following descriptions of the same event in different newspapers:
- On March 1st, a large group of extremist insurgents brought life in CITY to a grinding halt when they prevented any traffic across the main bridge. Police forces were quickly able to restore order and arrested over a dozen insurgents in the process.
- On March 1st, a large demonstration of anti-regime protestors blocked traffic across the main bridge in CITY. Police forces dispersed the demonstration after one hour and arrested over a dozen protestors.
Both sources contain roughly the same facts:
- Large gathering of people on March 1st
- Motive: Political
- Location: CITY, main bridge
- Activity: block traffic
- Police response: dissolve gathering, arrest >12 people
But the second report contains one additional fact: it states "one hour" as the time it took the police to resolve the gathering, whereas the first report states "quickly". And this is where description bias begins: is "one hour" = "quickly"? Or does using "quickly" make the police seem more competent? How the people are described is also obviously biased: "extremist insurgents" is a very different description than "anti-regime protestors". And more: "blocked traffic" is decidedly more neutral than "brought life to a grinding halt".
Naturally, these two examples are rather extreme to make a point, but depending on which outlets you contrast, they are not an exception. Yet, the question remains: as a researcher, what do you do? The first thing to do is remove all the value judgements – you can code "one hour" quantitatively, which you can't do for "quickly". And for contentious concepts, you'll need to build your own taxonomy of entities. What makes something an anti-regime protestor vs. an extremist insurgent in your book? And, while you're reading, you'll have to keep these definitions in mind.
As a non-researcher, one question you can ask yourself in your day-to-day life when reading the news to counter description bias is:
- Are there synonyms for the words used that are less loaded?
To sum up, we covered two biases that often influence the news we consume: Selection Bias and Description Bias. Selection Bias describes what gets reported in the first place, and Description Bias relates to how it gets reported. To counter both, you can ask yourself the following questions while reading the news:
- What is the likelyhood of this particular event being reported a year ago vs. now?
- What is the likelihood of this particular being reported a year from today vs. now?
- Are there other, similar sources that report the same thing?
- Are there synonyms for the words used that are less loaded?
If you want to dive deeper into how social scientists treat this issue, I highly recommend this article by Earl et al..
Related Topics
Join the Cortex Futura Newsletter
Subscribe below to receive free weekly emails with my best new content, or follow me on Twitter or YouTube.
Join and receive my best ideas on algorithms of thought, mental models and reading